Advancing Multi-omics Analysis by Co-localizing Data, Workflows, and Computation
The power – and increasing cost-effectiveness – of ‘omic technologies has provided groundbreaking insights into the underpinnings of biology and has revolutionized the process of target and drug discovery. A method like transcriptomics can provide a snapshot of genetic activity under certain conditions while proteomics can tell us which of those transcripts are being translated into proteins, providing a more detailed mechanism to understand the functional implications of post-translational modifications. Critically, while each of these methods provides incredible detail about specific levels of biology, they are just snapshots and provide little insight into the levels of biology further up or lower down the chain of complexity.
Ten years ago, multi-omic papers were few and far between [1] (Fig 1.). These days, multi-omics studies abound, with a 2022 meta-GWAS boasting a minimum of 4 different ‘omic technologies to functionally rank Alzheimer’s risk genes [2]. This shift to a multi-omic approach is a critical step in advancing our ability to understand and treat the complex underlying biology of human diseases. One disease case where this shift is necessary is Alzheimer’s.
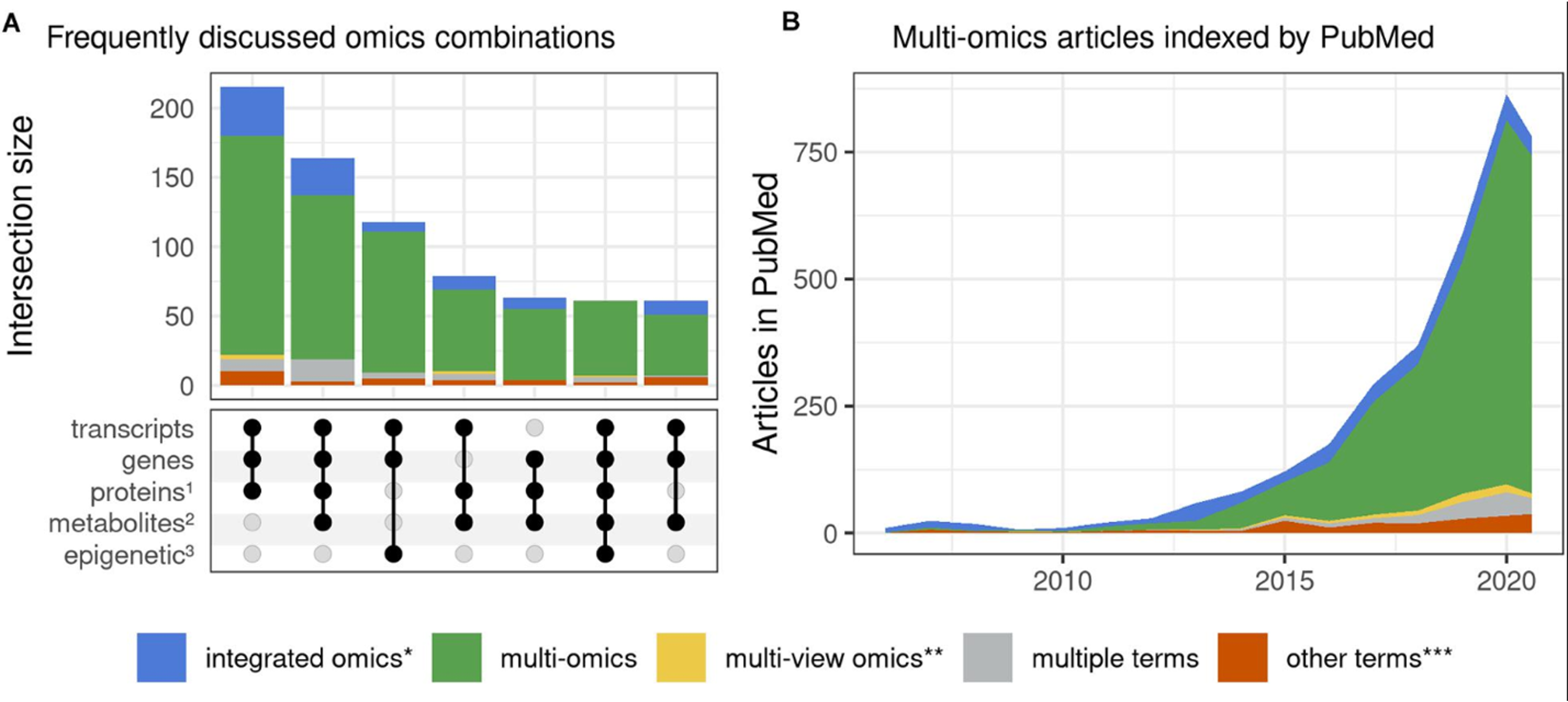
In the case of Alzheimer’s Disease, the focus of my graduate studies, a series of meta-GWAS published since 2019 have led to a veritable explosion of genome-wide significant risk variants [2-4]. However, the disease is not just the variant and there are many layers of biology compounding or protecting against that deleterious mutation all converging to produce a cognitively normal individual or someone suffering from advanced Alzheimer’s to every cognitive phenotype in between. The only way to understand the disease implications of a risk variant, is to study it in the context of the other layers of biology contributing to the disease status.
But it is a long and arduous road from knowing that you need to take a multi-omic approach to studying your disease to implementing that approach in a user-friendly, scalable, and cost-effective way. At least it was for me. Identifying the data that I needed, installing all the tools to process and analyze it – and reinstalling different versions to make sure all the software functioned together – and finally writing and implementing the code to do all the analysis took me a year of long weeks and sleepless nights of work. As an academic exercise, the time and effort I put into the project was well worth it. For pharmaceutical companies, on the other hand, a year of extra work could mean the difference between a groundbreaking drug going to market a year before or a year after the closest competition. Again, Alzheimer’s is a great example with both Biogen and Eli Lilly releasing their monoclonal amyloid beta antibody treatments, Leqembi and Donanemab respectively, in the past year.
To make up that year and gain a competitive advantage all while furthering our collective understanding of disease biology, pharmaceutical companies and academic research labs need to embrace the power of a complete bioinformatics solution like the Velsera Seven Bridges Platform. First, the Platform is entirely data-type agnostic allowing researchers to design the most complex of multi-modal data analyses in one convenient cloud-based location. If you need access to even more data, the Seven Bridges Platform enables easy access to myriad public datasets like TCGA, TARGET, CPTAC, and the CCLE. Controlled access data in these repositories can also be accessed through the Seven Bridges Platform by connecting your approved dbGaP credentials. If the data that you need isn’t immediately available, our team of scientists can help find the right datasets for your project and help get them connected to the platform.
If you’re working solely with in-house multi-modal data stored in the cloud, you can bring the scalable compute power of the Seven Bridges Platform right to where your data lives by connecting your AWS, Google Cloud Storage, and Azure buckets directly to the Platform. Not only does this do away with the headache of transferring your files from a local machine to an HPCC for primary analysis and then back for secondary and tertiary analysis, but it ensures that you maintain absolute control over your data in your own storage solution. As an added benefit, the attached RStudio and JupyterLab instances allow for tertiary analysis in your preferred development environment and our prebuilt TensorFlow environment provides easy access to powerful machine learning tools used in multi-modal image analysis.
Furthermore, with the Seven Bridges Platform, gone are the days of wrangling package dependencies to ensure that your entire workflow consisting of 1000+ lines of code doesn’t collapse around you because one minor package needed an update. All of our 775+ tools and workflows in our public apps gallery are actively maintained by our exceptional bioinformaticians. Additionally, each one is wrapped in Common Workflow Language, which is a powerful open-source framework to ensure that distinct tools can easily work together, a necessity for a multi-omic workflow. This also ensures that every workflow will run the same each time it is used, ensuring that your results are robust and reproducible.
While the Seven Bridges Platform would have been convenient for me as I slogged through my dissertation project, it is a necessity for pharmaceutical labs developing the treatments and cures of tomorrow. To advance scientific progress, the Seven Bridges Platform makes it as easy as a click of a button to implement a multi-omic workflow, while breaking down data silos that might have otherwise stopped a research program in its tracks. Access to the Seven Bridges Platform in graduate school would have saved me three to four months of headaches. In the realm of drug development, three to four months could mean extending or saving a patient’s life with a breakthrough treatment. The days of homegrown workflows and cobbled together datasets are well behind us. If the life science industry is going to truly deliver on the promise of precision medicine that has been teased since the end of the Human Genome Project, it needs to whole-heartedly embrace the value of multi-omic analyses and the power of cloud-based bioinformatics platforms.
Want to learn more?
Interested in learning more about the importance of multi-modal analysis and imaging in precision medicine? Join us on August 16 for our webinar “Advancing Precision Medicine: Multi-Modal Analysis and Imaging for Enhanced Diagnosis and Treatment.” Register here to learn from Dr. Surya Saha, a Technical Program Manager in the Scientific Programs team at Velsera. https://26660662.hs-sites-eu1.com/multi-modal-analysis-and-imaging-for-enhanced-diagnosis-and-treatment
References:
- Krassowski, M., Das, V., Sahu, S. K., & Misra, B. B. (2020). State of the Field in Multi-Omics Research: From Computational Needs to Data Mining and Sharing. Frontiers in genetics, 11, 610798. https://doi.org/10.3389/fgene.2020.610798
- Bellenguez, C., Küçükali, F., Jansen, I. E., Kleineidam, L., Moreno-Grau, S., Amin, N., Naj, A. C., Campos-Martin, R., Grenier-Boley, B., Andrade, V., Holmans, P. A., Boland, A., Damotte, V., van der Lee, S. J., Costa, M. R., Kuulasmaa, T., Yang, Q., de Rojas, I., Bis, J. C., Yaqub, A., … Lambert, J. C. (2022). New insights into the genetic etiology of Alzheimer’s disease and related dementias. Nature genetics, 54(4), 412–436. https://doi.org/10.1038/s41588-022-01024-z
- Jansen, I. E., Savage, J. E., Watanabe, K., Bryois, J., Williams, D. M., Steinberg, S., Sealock, J., Karlsson, I. K., Hägg, S., Athanasiu, L., Voyle, N., Proitsi, P., Witoelar, A., Stringer, S., Aarsland, D., Almdahl, I. S., Andersen, F., Bergh, S., Bettella, F., Bjornsson, S., … Posthuma, D. (2019). Genome-wide meta-analysis identifies new loci and functional pathways influencing Alzheimer’s disease risk. Nature genetics, 51(3), 404–413. https://doi.org/10.1038/s41588-018-0311-9
- Wightman, D. P., Jansen, I. E., Savage, J. E., Shadrin, A. A., Bahrami, S., Holland, D., Rongve, A., Børte, S., Winsvold, B. S., Drange, O. K., Martinsen, A. E., Skogholt, A. H., Willer, C., Bråthen, G., Bosnes, I., Nielsen, J. B., Fritsche, L. G., Thomas, L. F., Pedersen, L. M., Gabrielsen, M. E., … Posthuma, D. (2021). A genome-wide association study with 1,126,563 individuals identifies new risk loci for Alzheimer’s disease. Nature genetics, 53(9), 1276–1282. https://doi.org/10.1038/s41588-021-00921-z