Circulating Tumor DNA Analysis on the Seven Bridges Platform
Next Generation Sequencing (NGS) has brought significant improvements in all areas of biotechnology and healthcare — and cancer screening is no exception. The latest game-changer is liquid biopsy, a novel and challenging NGS application. By directly measuring and analyzing circulating tumor DNA (ctDNA) in blood, liquid biopsy is a promising, non-invasive method for cancer diagnostics and disease monitoring which improves upon the classical approach of following biomarkers with moderate specificity.
However, sequenced ctDNA is limited in both quality and quantity, as it is usually represented in fraction < 1.0% of total cell free DNA in human blood1. While these obstacles are addressed through advances in the biochemical procedures of library preparation, bioinformatic analyses must also be adapted to take advantage of such improvements. We are excited to announce that Seven Bridges has developed a dry-bench procedure for ctDNA analysis with significantly improved sensitivity through innovative processing steps developed internally by our ctDNA team and well-known public tools. Figure 1 represents the schema for the combined targeted and whole exome sequencing (TRS/WES) workflow recently developed by the Seven Bridges bioinformatics ctDNA team.
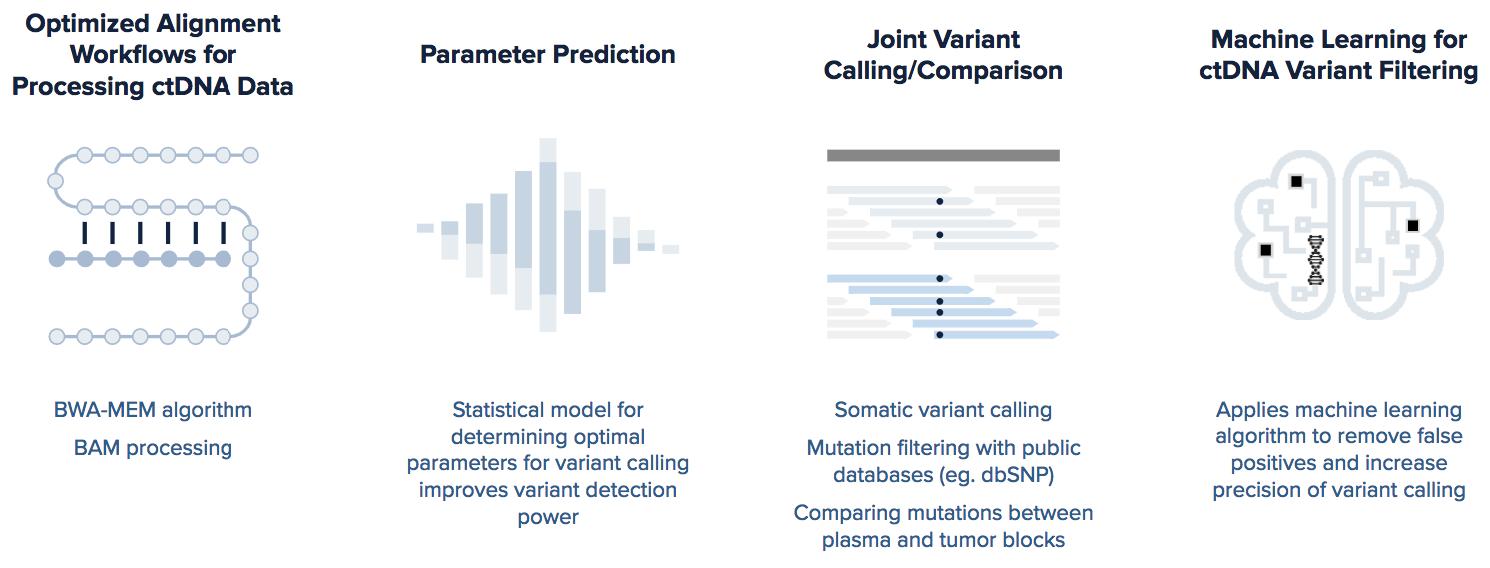
The analysis of ctDNA is challenging due to very low sample purity and the use of a single somatic caller underperforms even with parameter tuning. We identified that the results from the single caller usually lacked high quality results, especially in terms of sensitivity. As such, our first improvement was to combine the results from several different somatic variant callers to improve performance metrics.
A major challenge in analyzing ctDNA is cell diversity in primary tumors and potential cell diversity in metastatic sites. To address this challenge, we developed an approach for optimizing somatic variant calling parameters based on individual sample characteristics (e.g. coverage, sequencing error rate, capture kit, etc.). Specifically, we developed a tool which mathematically models sample characteristics and suggests the parameters to use for calling where applicable. Not only did this dramatically improve recall, it also significantly advanced precision in further analysis.
The final step in our workflow is a machine learning approach that filters out likely false positive variants based on specific variant attributes using a model trained on publicly available datasets2-5. As Figure 2 below indicates, improving accuracy in a 4-step analysis increased sensitivity by approximately 30% and precision by 55% for a targeted sample4.
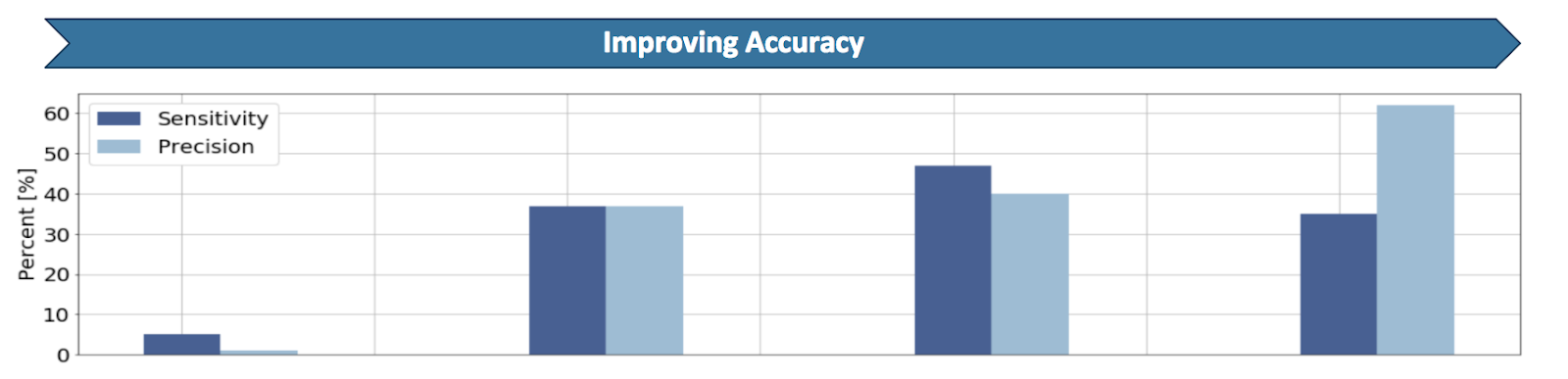
Our analyses were performed on combined TRS/WES datasets which include 52 samples with various cancer types, such as leukemia, sarcoma, breast cancer, lung cancer in different stages2-5. We found that parameter prediction is the most time-consuming part of the analysis due to its thorough examination of BAM files and the prediction of optimal parameters for calling. Although variant calling with multiple different callers also takes a substantial portion of the analysis in the regular run, we managed to optimize this by designing the workflow to execute all callers in parallel.
The approximate cost and runtime of the analysis on our training datasets in relation to input size are depicted in Figure 3 below. Based on the training datasets, a workflow executed on a single TRS sample of average input size of 3GB completes in 1.3 hours and costs $0.51, while for a single WES sample of 17.5GB average input size, cost, and execution time are $1.49 and 3.75 hours respectively. Please note that these numbers are estimates based on our training dataset and may vary for other samples. However, depending on the sample, further computing and workflow optimizations may be available.
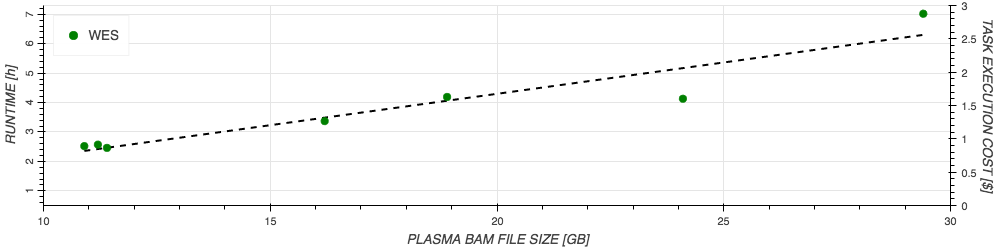
We have developed and validated our analysis on a variety of publically available datasets, and we are determined to continue in this manner in order to demonstrate that our method performs well. Our goal is to tackle the diversity of ctDNA datasets by finding different cases and adapting steps to the disease stage and progression, including new features based on the incoming data. All segments of the model will live and evolve with the methodology of our dedicated ctDNA team.
We welcome the opportunity to collaborate with experts and teams around the world to improve our workflows and to evaluate our workflow performance with emerging datasets that represent the range of cancer types and stages. Please contact us for more information about our liquid biopsy workflow or about collaborations.
Works Cited
1. Luis A. Diaz Jr and Alberto Bardelli Liquid Biopsies: Genotyping Circulating Tumor DNA. Journal of Clinical Oncology 2014 32:6, 579-586
2. Yeh, P. et al. Circulating tumour DNA reflects treatment response and clonal evolution in chronic lymphocytic leukaemia. Nat. Commun. 8, 14756 doi: 10.1038/ncomms14756 (2017).
3. Butler TM, Johnson-Camacho K, Peto M, et al. Exome Sequencing of Cell-Free DNA from Metastatic Cancer Patients Identifies Clinically Actionable Mutations Distinct from Primary Disease. Richards KL, ed. PLoS ONE. 2015;10(8):e0136407. doi:10.1371/journal.pone.0136407.
4. Newman AM, Bratman SV, To J, et al. An ultrasensitive method for quantitating circulating tumor DNA with broad patient coverage. Nature medicine. 2014;20(5):548-554. doi:10.1038/nm.3519.
5. Mao, Xiaowei et al. Capture-Based Targeted Ultradeep Sequencing in Paired Tissue and Plasma Samples Demonstrates Differential Subclonal ctDNA-Releasing Capability in Advanced Lung Cancer. Journal of Thoracic Oncology, Volume 12, Issue 4, 663 – 672.